Melon Intel is an app concept that uses machine learning to analyze a watermelon for ripeness…
The Problem:
Is the watermelon you picked up actually ripe?
This idea was partly inspired by an old podcast segment from CBC, Spark #343, The Shazam of Machines, where specialized sensors and Machine Learning technology from Augury, are used to monitor a machine’s sound and vibration 24/7 and will find and predict problems before they become disruptive failures.
Augury counts Pepsico as a client, using their tech to keep their snack food production empire humming along. This application of continuously monitoring sound and vibration appealed to me, stuck in my mind over the years for this potential to find problems before failure that we can’t see, and also as a tangible use case widely applicable for the IOT future that has not quite arrived, but I digress.
Enter the watermelon, the enigmatic melon which could be deliciously sweet and juicy or past due, gritty and pale or worse inside. Judging from the countless articles on the subject of how to determine a melon’s ripeness, this is a pain point of summer grocery shopping where strong feelings of buyer remorse can set in.
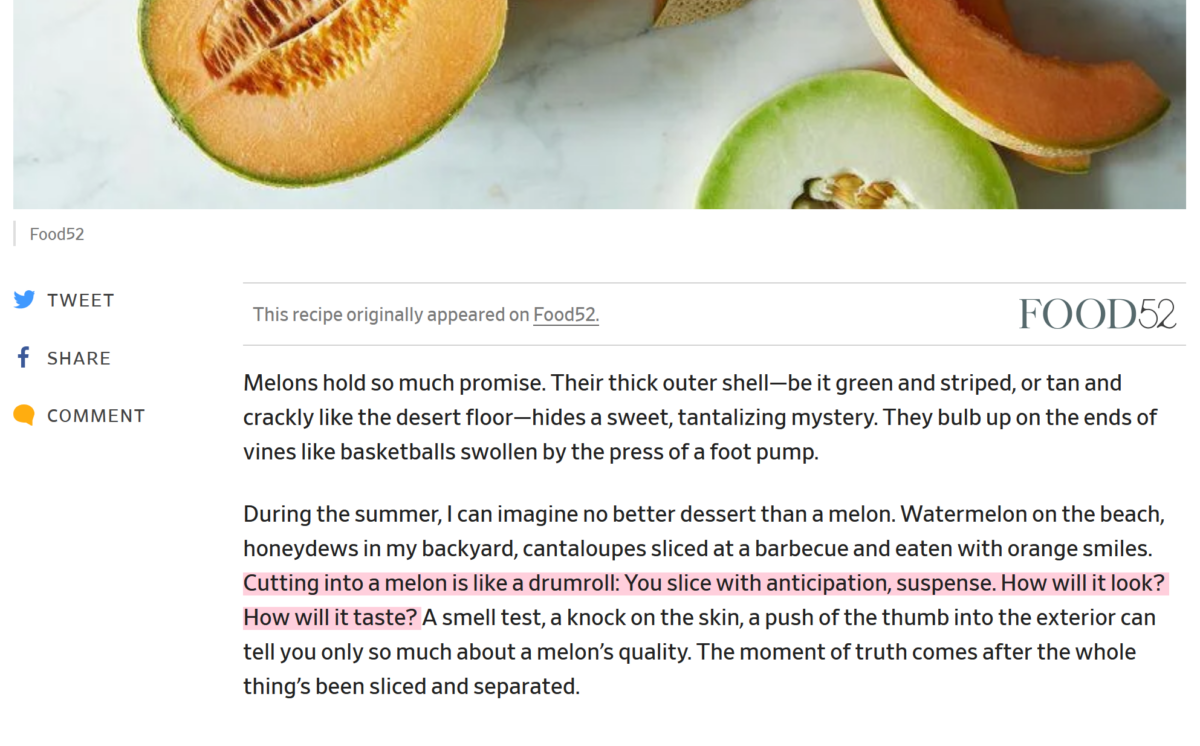
If you happen on a bad melon, Slate has a few ways you could save it, namely a pinch of salt.
Desk Research
Digging further into search analytics shows the relative trends for users looking for tips to pick a ripe watermelon. Google shows how the search volume is seasonal, focused in the summer and competes well with other fruits.
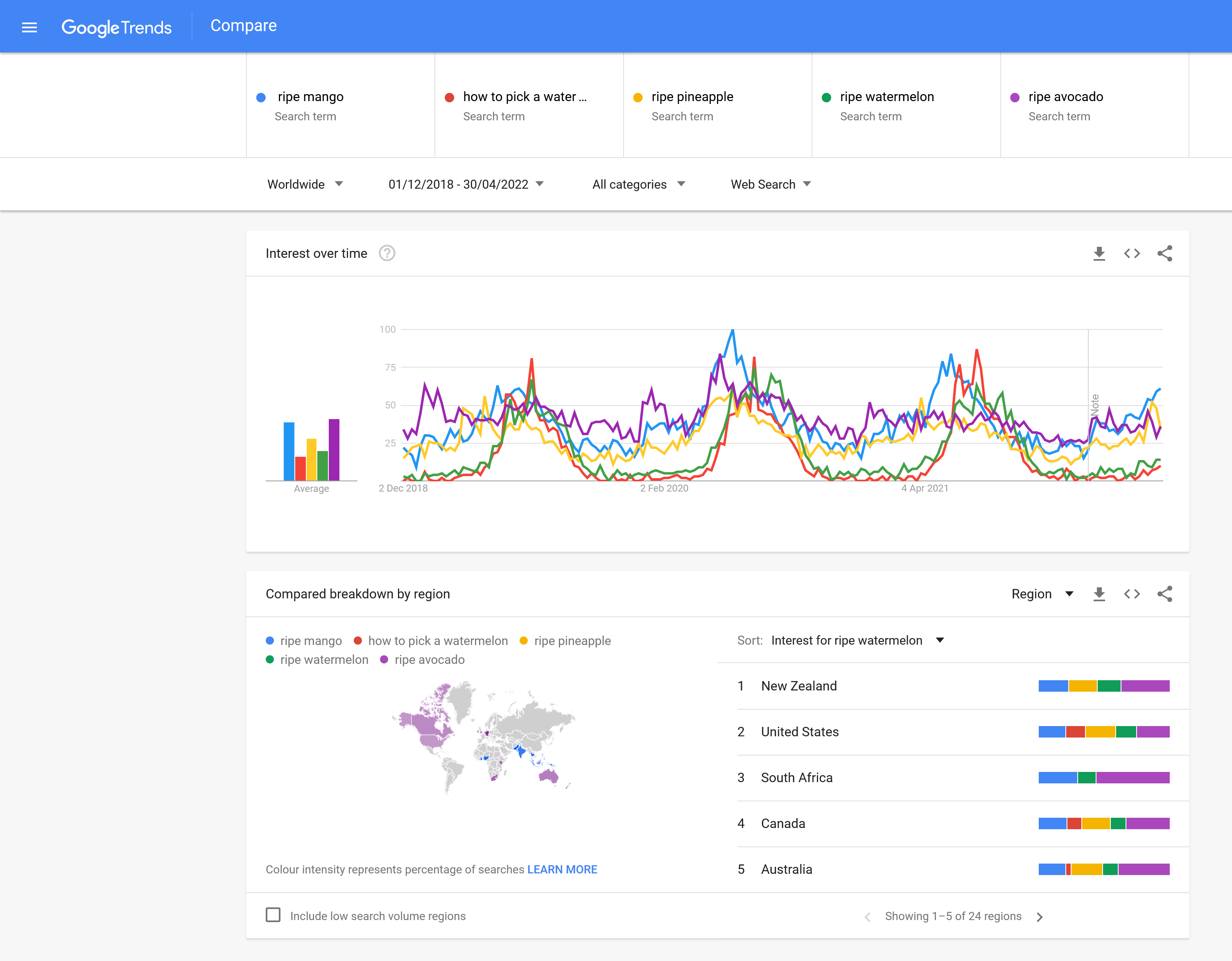
By making use of a tool like WordStream, I could further qualify the relative volume of searches; while Google Trends shows “ripe watermelon” is a good keyword, the phrase “how to pick a watermelon” is shown ranking in 40,000 queries monthly to just 6,600! These numbers taken together paint a ballpark picture of the user interest out there in finding a ripe melon among other fruits; again you can see in 2021 “how to pick a watermelon” peaks as high as mango and doubles over [sustained/consistent] avocado queries.
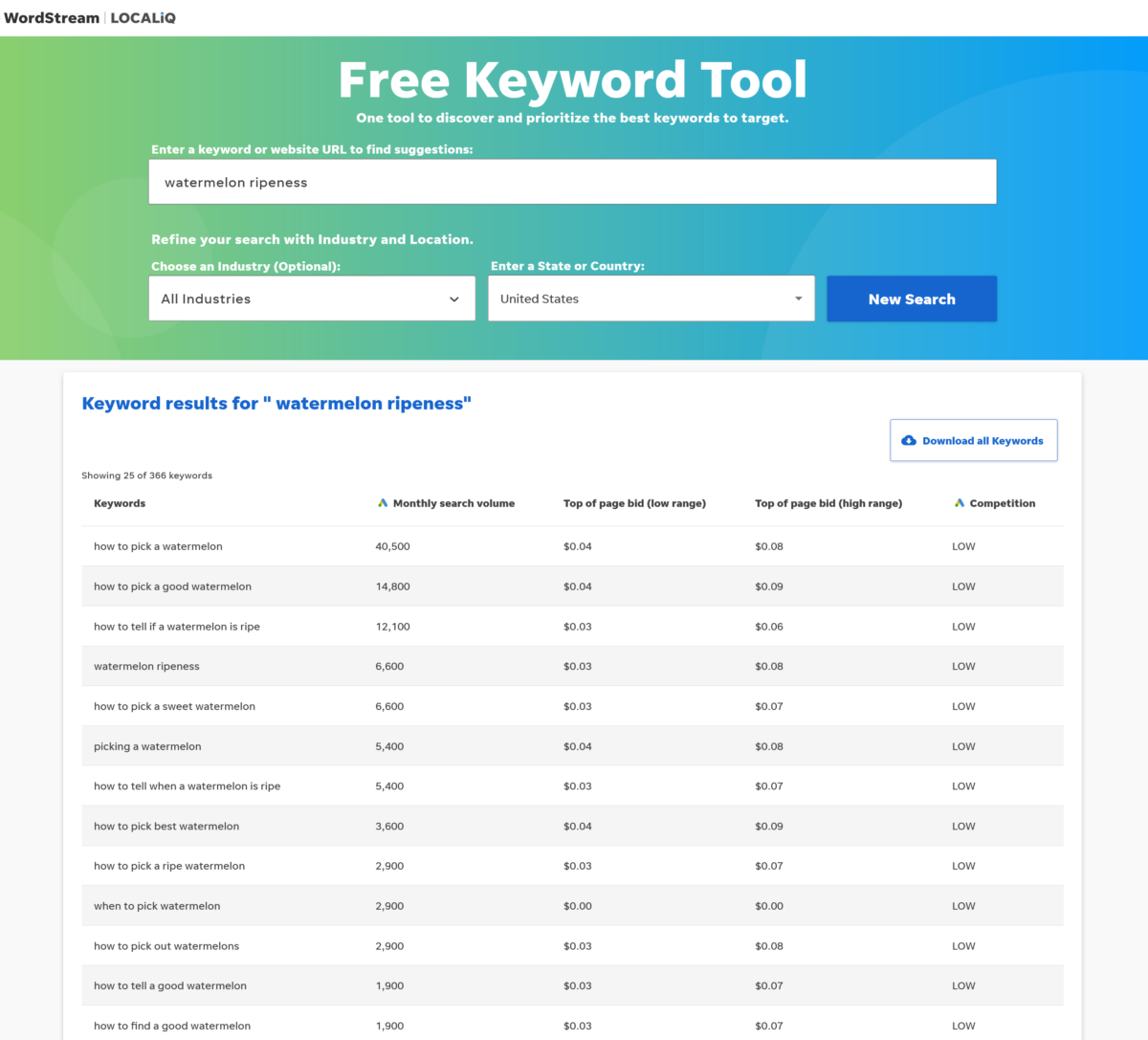
With this specific use case established, the potential for this app is to help the shopper make a more informed decision in store before buying a watermelon. A question that comes naturally out of this query exploration and worth a real survey, is the purchase intent: “How many shoppers decide to not bother with choosing a watermelon based on the unknown factor of whether it is sweet or not?”
Exploration
As an app, Melon Intel would essentially tackle that uncertainty for the user, and simplify the [assumed] burden of choosing one in a cheerful way that focuses on quickly tapping a melon with your phone.
This main function as both a proof of concept and technology means the ideal initial user flow is quite simple and should actively guide the user to tap their phone on the melon, providing visual feedback and then a grade of ripeness.
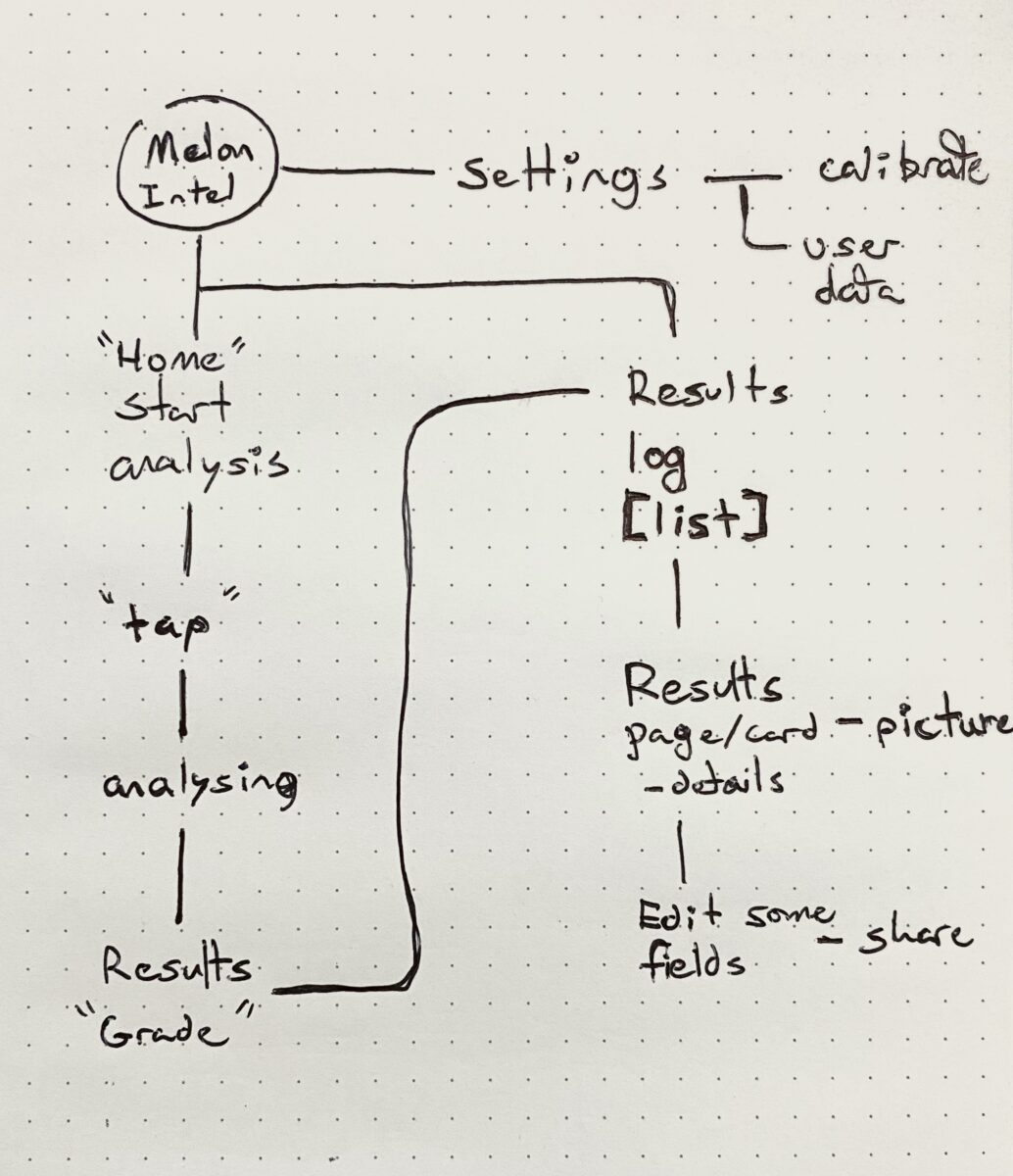
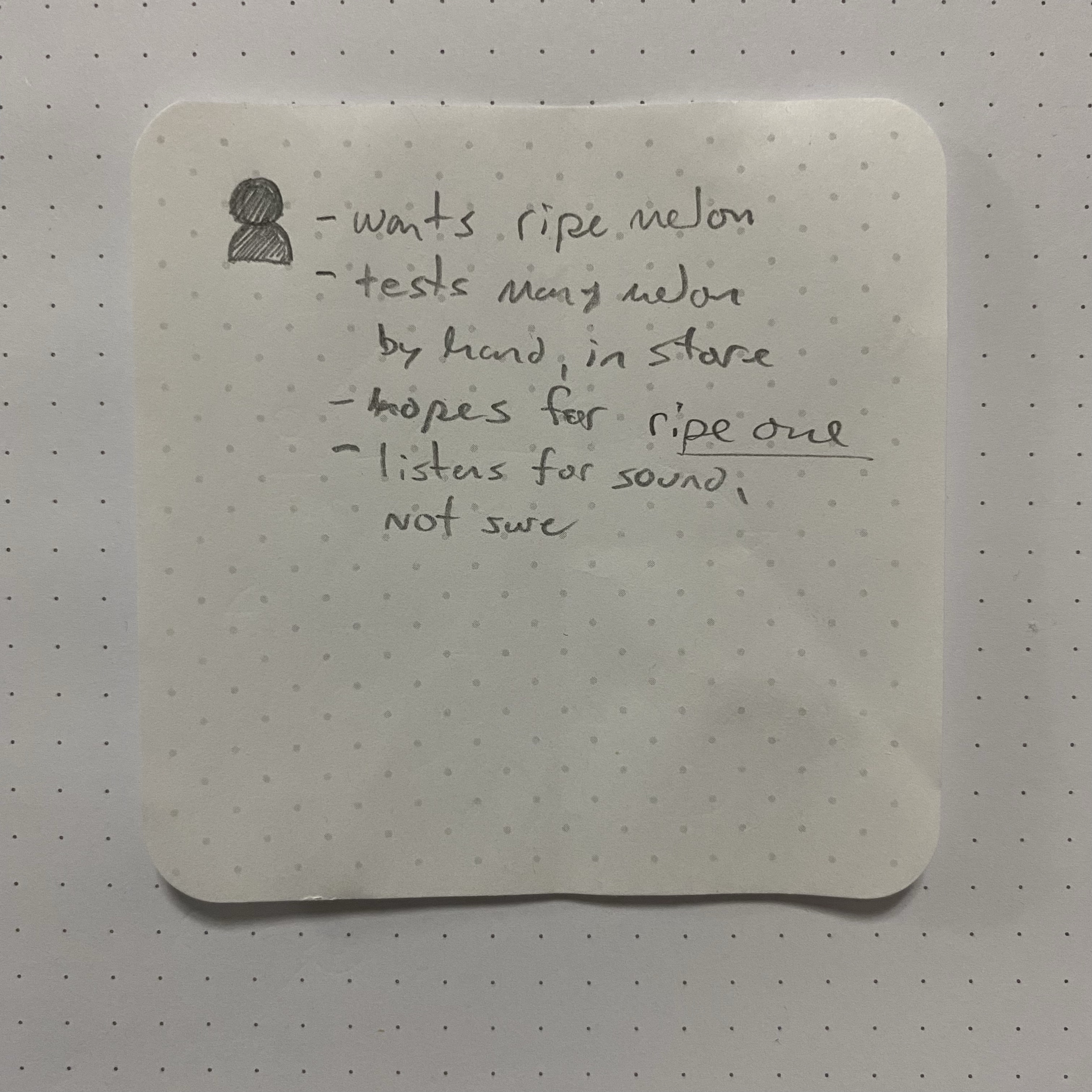
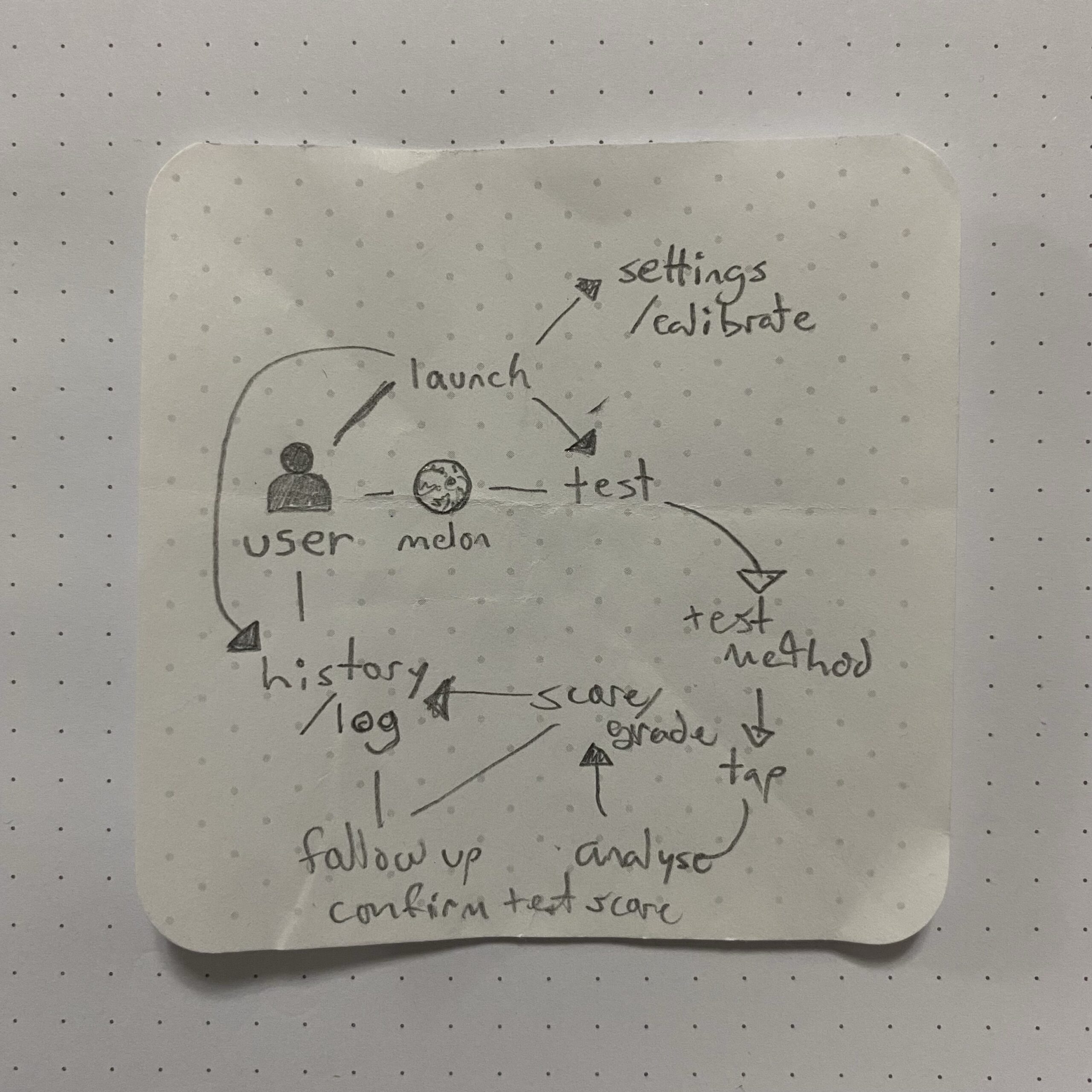
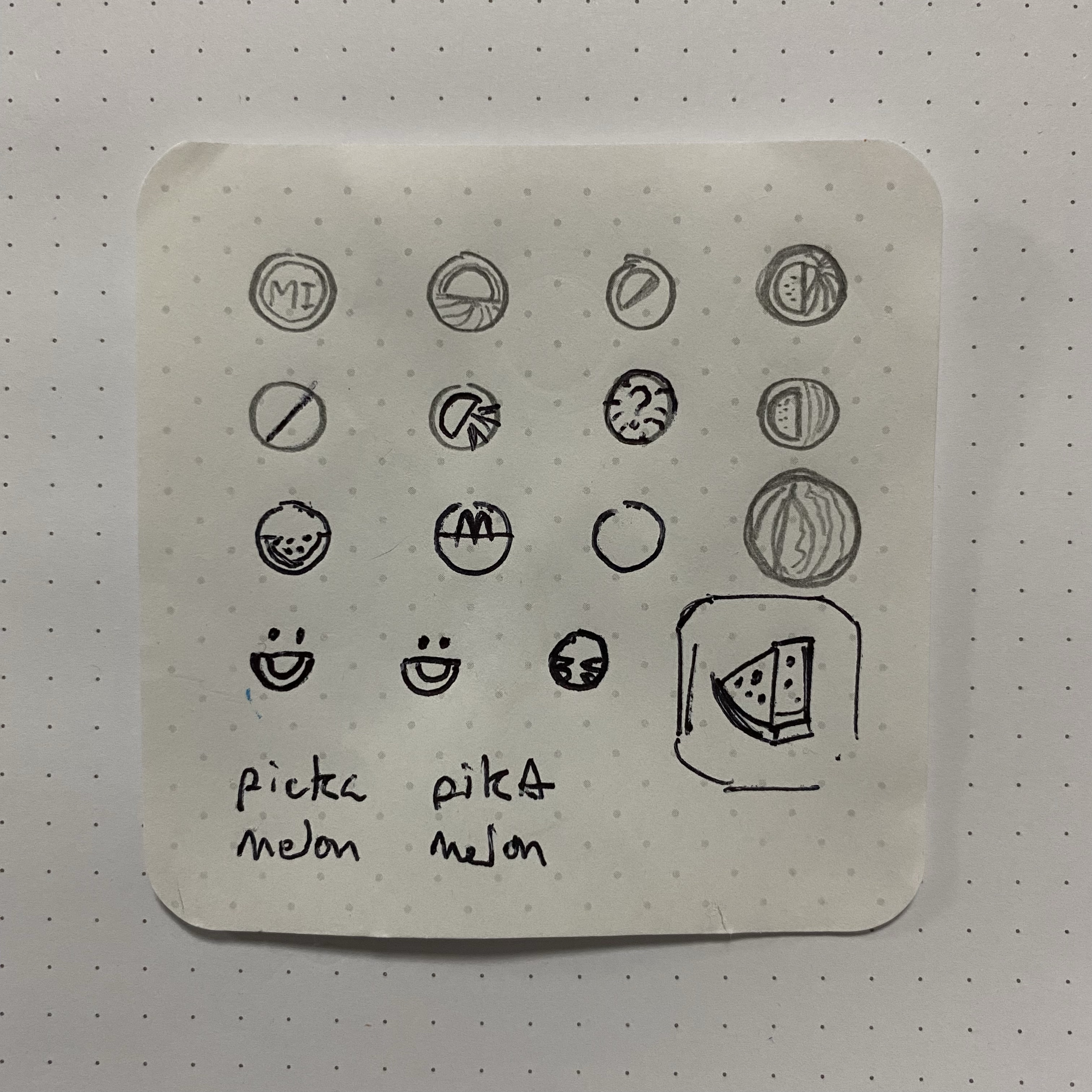
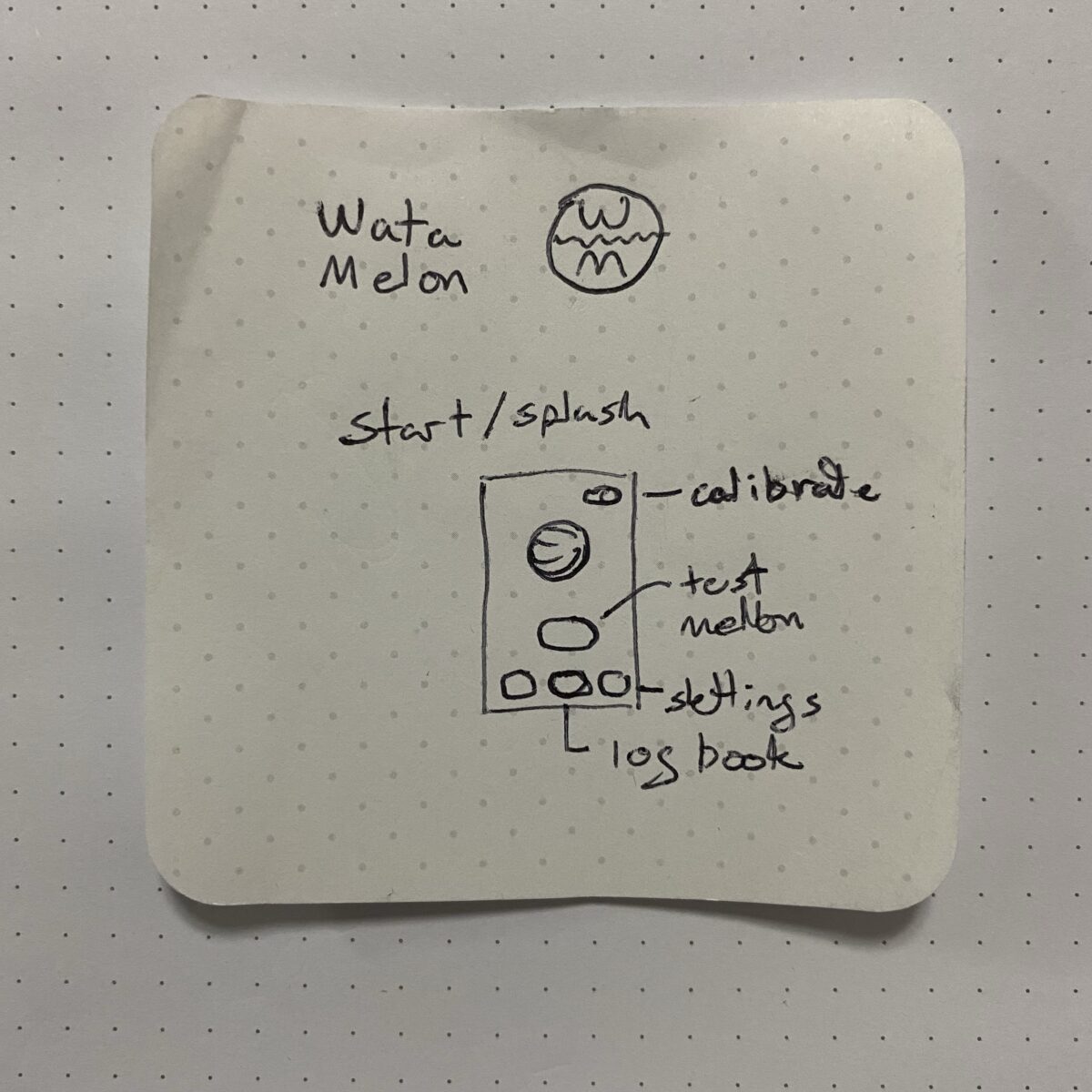
To go back to the beginning about sensors analyzing machined for problems; here the case is a specialized Machine Learning algorithm is trained to analyze data captured of sound + echo and the impact of tapping your smartphone twice on the rind.
I postulate, by using both microphone and accelerometer data of modern smartphones, Melon Intel can assess a grade of ripeness to the melon in question; and potentially demystifying the melon enough to even bump sell through!
Outcome: Mid-Fi Mock Up
The concept app is rendered here in Figma, to demonstrate the user flow of testing a melon in hand, as well as retrieving a record of the tests with date, time etc.
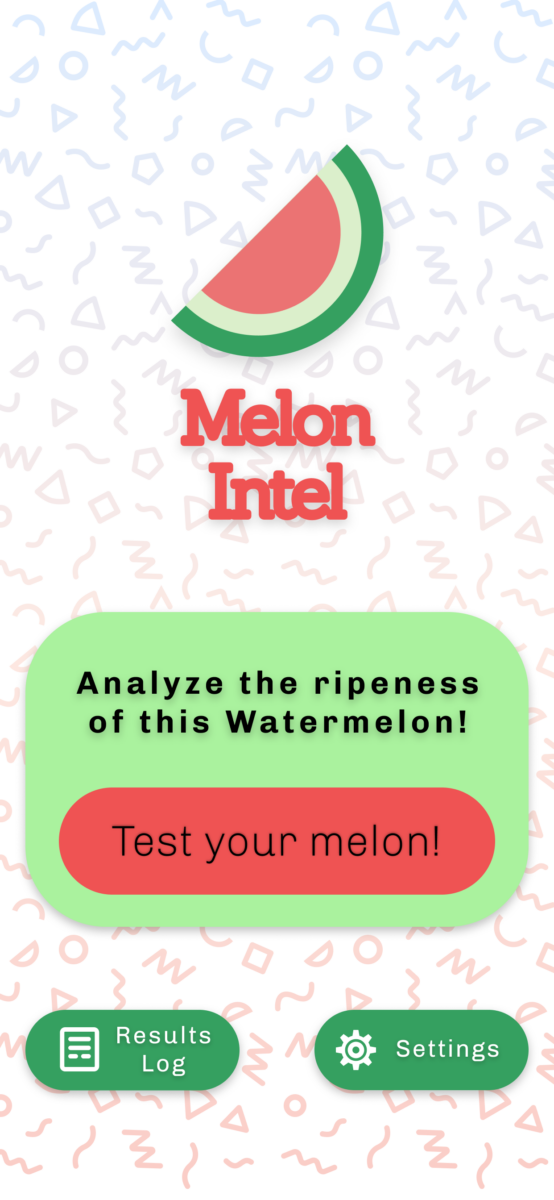
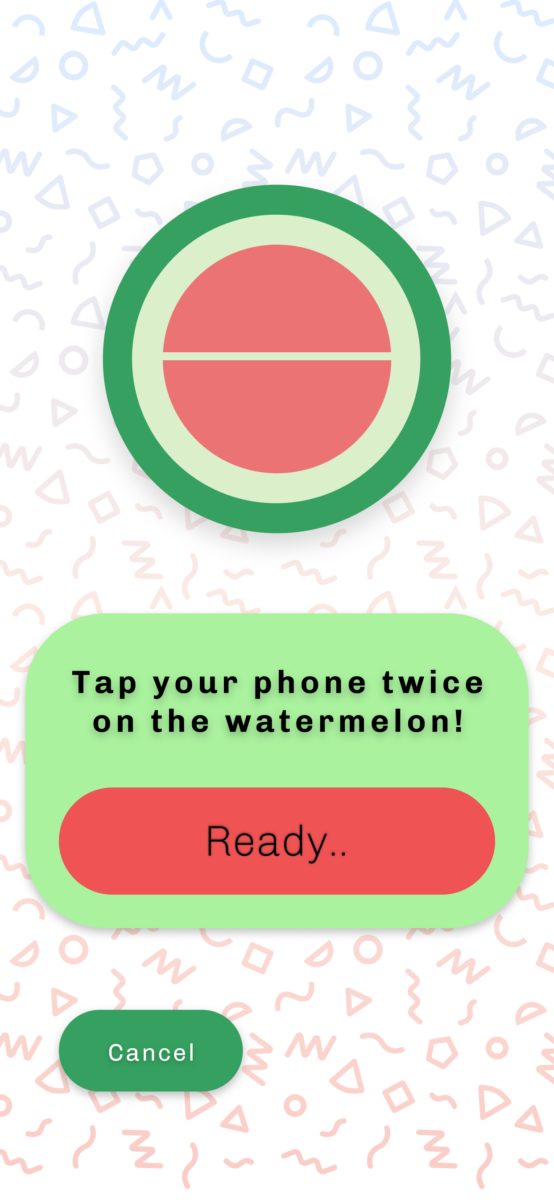
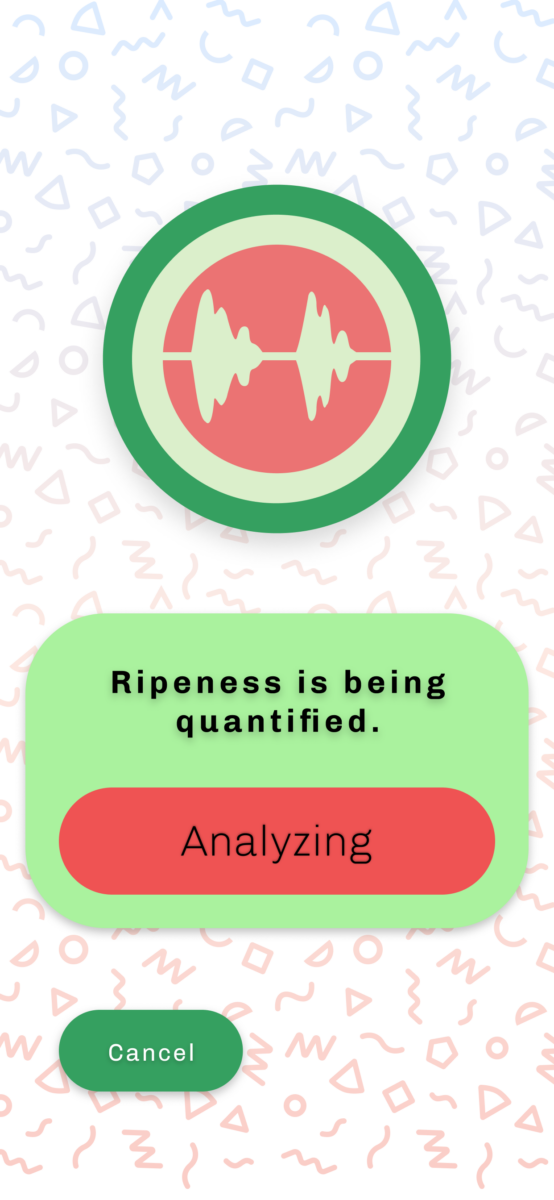
You must be logged in to post a comment.